TOP 9 AI Tools for Data Analytics in 2025
In the ever-evolving landscape of data analytics, artificial intelligence (AI) has emerged as a powerful ally for data professionals. The year 2023 brings forth a new wave of AI for data analytics that are transforming the way data is analyzed, and insights are gleaned. This article delves into the realm of AI data analytics, exploring the role of an AI data analyst and presenting a comprehensive list of the top 9 AI data analysis tools that are making waves this year.
From PDF summarizers to generative ai for data analytics platforms, we will uncover their main features, pros, and cons, shedding light on how these tools can elevate data analytics to new heights. Furthermore, we will guide you through how to harness AI for data analysis and address common questions related to AI in data analytics.
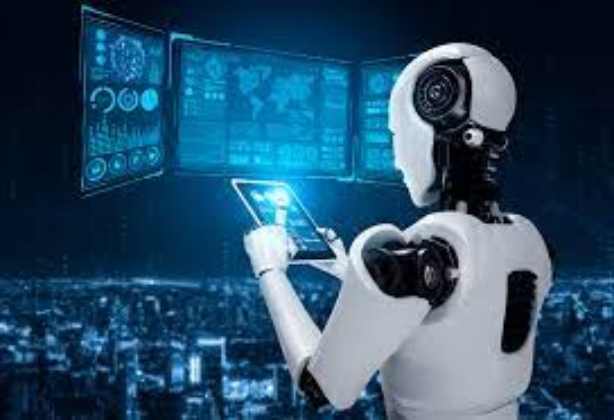
Catalogs:
Part 1: What Does an AI Data Analyst Do?
Before we dive into the AI tools themselves, it's crucial to understand the role of an AI data analyst. An AI data analyst is a professional who leverages AI-powered tools and techniques to collect, process, analyze, and interpret analytical skills for ai and data science. These experts utilize AI algorithms to identify patterns, generate insights, and make data-driven decisions.
Part 2: What Are the Top 9 AI Data Analysis Tools?
Top 1: Tenorshare AI - PDF Tool
Tenorshare AI - PDF Tool is a leading AI tools for data analytics designed to extract and summarize information from PDF documents swiftly. This powerful tool stands out for its user-friendly interface, capable of handling PDFs of various lengths and types. Users can save precious time by quickly extracting essential information from PDFs and even asking the tool questions related to the document. Its intelligent summary formation offers an instant overview of the entire document. However, it may not provide precise answers for content beyond the 10th page.
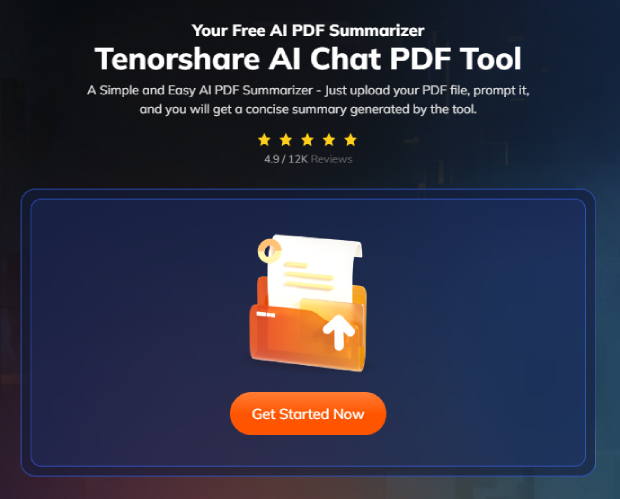
Main Features
- Extracts information from PDFs efficiently.
- User-friendly chat interface.
- Summarizes PDFs in minutes.
- Multilingual answers based on uploaded PDF language.
- Web-based tool, no downloads required.
Pros
- User-friendly and quick start.
- Multilingual support.
- Humanized replies for a natural interaction.
- Instant document overview.
- Web-based convenience.
Cons
- Less precise for content beyond the 10th page.
How to Use Tenorshare AI - PDF Tool
Step 1: Visit the website and upload your PDF files.
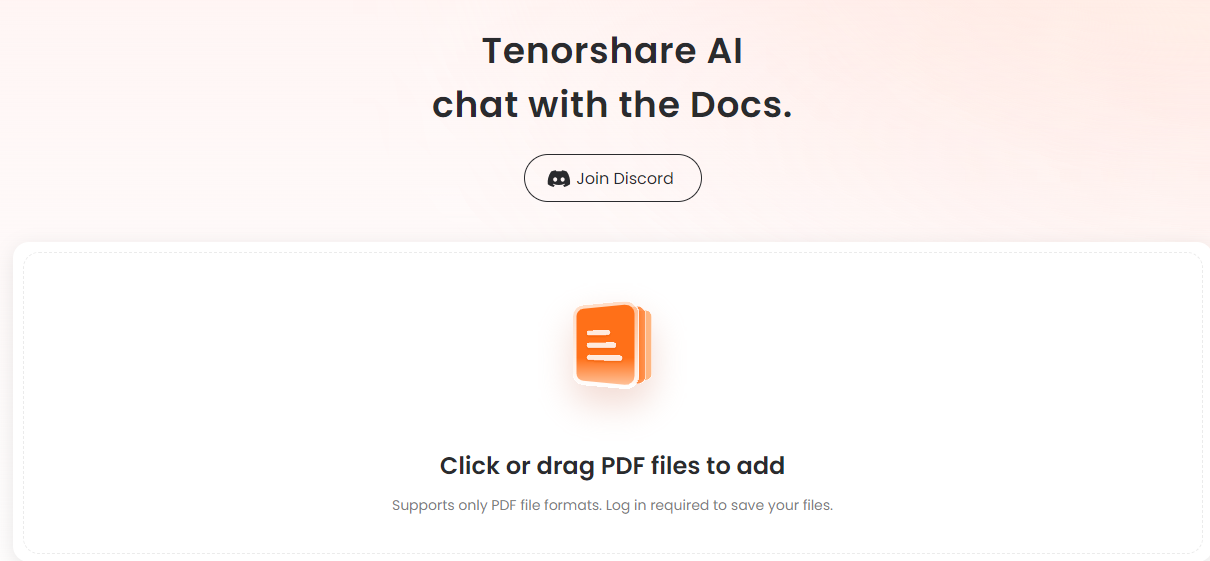
Step2: Create an account and log in.
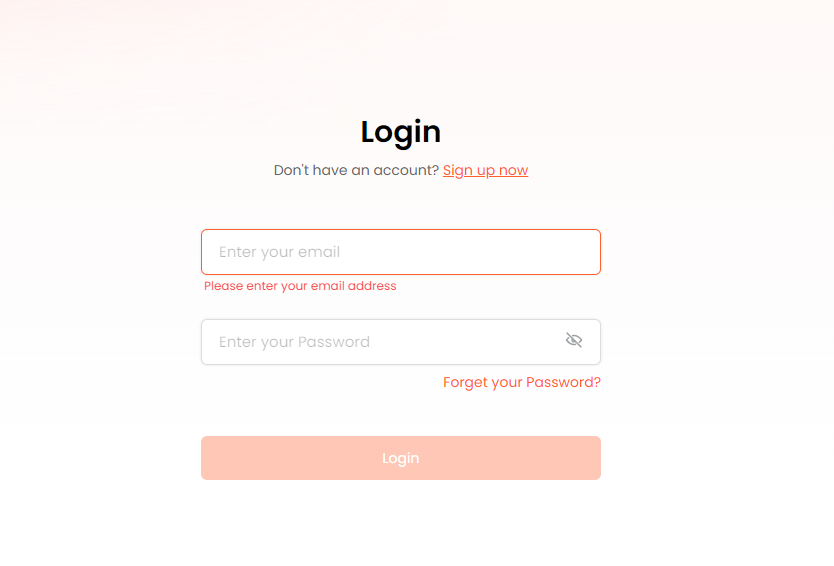
Step 3: Ask questions or request PDF summarization to analytical skills for ai and data science pdf.
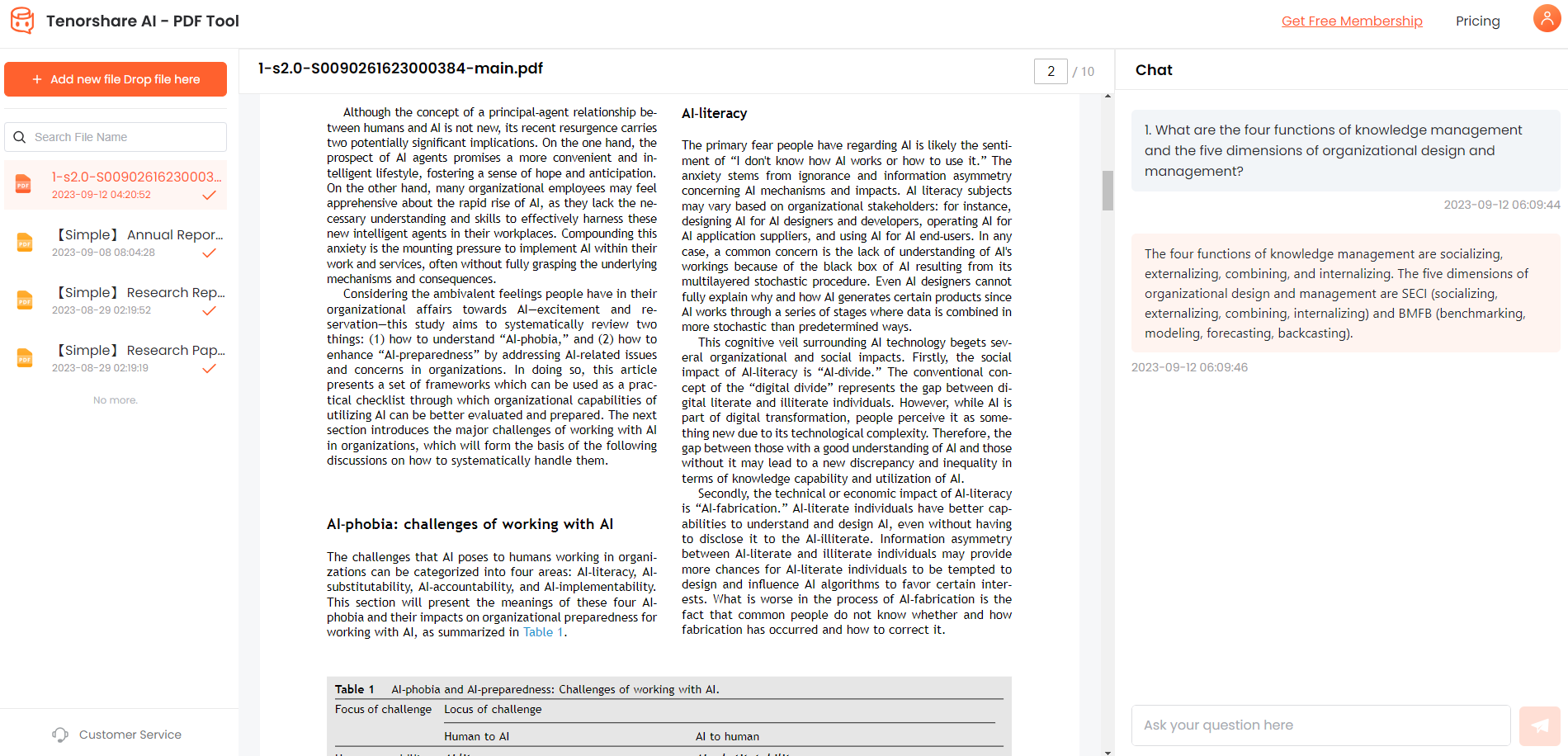
Top 2: RapidMiner
RapidMiner is a versatile data science platform that employs AI and machine learning for data analysis. It offers a wide range of features for data preprocessing, modeling, and evaluation.
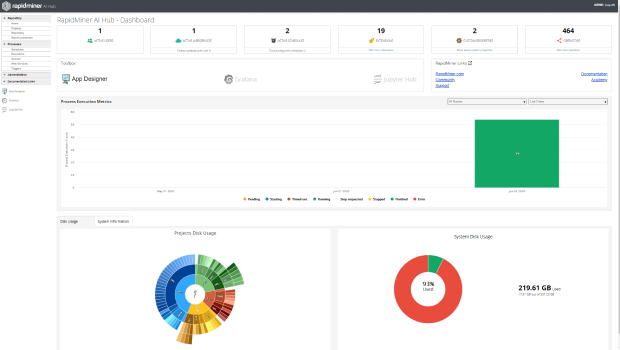
Main Features
- Intuitive visual interface.
- Supports various data sources.
- Advanced machine learning algorithms.
- Automated machine learning (AutoML) capabilities.
Pros
- User-friendly, ideal for beginners.
- Strong support for machine learning.
- Integration with popular data storage and analytics tools.
- AutoML streamlines model building.
Cons
- Advanced features may require a learning curve.
- Limited free version.
User Experience
Users appreciate RapidMiner's user-friendly interface and robust machine-learning capabilities. It simplifies complex data analysis tasks.
Top 3: KNIME
KNIME is an open-source platform for AI for data analytics, reporting, and integration. It is known for its flexibility and extensibility, making it suitable for various data analysis tasks.

Main Features
- Modular workflow design.
- Large collection of data analysis nodes.
- Integration with external tools and libraries.
- Scalable for big data.
Pros
- Open-source and community-driven.
- Versatile and extensible.
- Support for various data types.
- Integration with external tools.
Cons
- It may require technical expertise.
- The steeper learning curve for beginners.
User Experience
Users find KNIME powerful and versatile, but note that it might be intimidating for those new to data analytics.
Top 4: Tableau
Tableau is a renowned data visualization tool that combines AI and interactive features to create stunning data dashboards and reports.
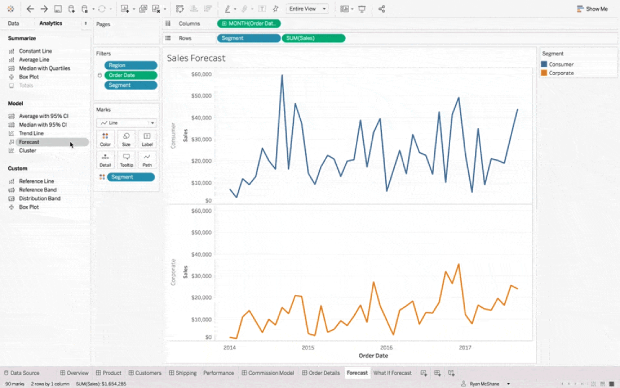
Main Features
- Drag-and-drop interface.
- Real-time data connections.
- AI-powered insights.
- Sharing and collaboration features.
Pros
- Intuitive and easy to use.
- Powerful visualization capabilities.
- Real-time data connections.
- Collaboration and sharing options.
Cons
- Pricing may be prohibitive for some.
- Advanced features require training.
User Experience
Tableau is praised for its simplicity and ability to create captivating visualizations. It's favored among data professionals for its interactive and AI-driven insights.
Top 5: Google Cloud AutoML
Google Cloud AutoML is a cloud-based AI platform that enables users to build and deploy custom machine learning models with ease.
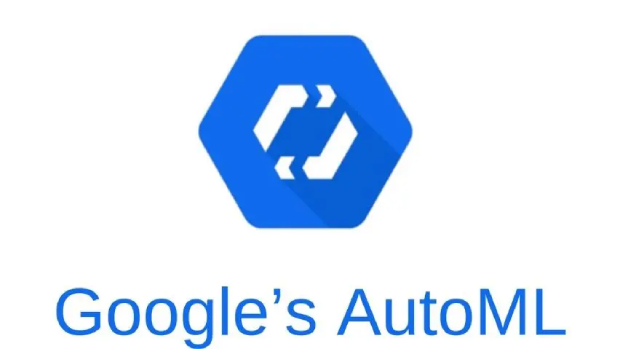
Main Features
- AutoML capabilities for various tasks.
- Pretrained models are available.
- Scalable and secure.
- Integration with other Google Cloud services.
Pros
- User-friendly, no coding required.
- Robust model customization.
- Scalable and cloud-based.
- Integration with Google Cloud services.
Cons
- Costs may escalate with extensive usage.
- Limited to Google Cloud ecosystem.
User Experience
Google Cloud AutoML is appreciated for its simplicity and the ability to create custom machine-learning models without extensive coding knowledge.
Top 6: Microsoft Azure Machine Learning
Microsoft Azure Machine Learning is a cloud-based platform that offers comprehensive tools for building, training, and deploying machine learning models.

Main Features
- End-to-end machine learning workflow.
- Integration with popular data science tools.
- Automated machine learning.
- Scalable and secure.
Pros
- Seamless integration with Azure services.
- User-friendly interface.
- Automated machine learning options.
- Scalable and secure.
Cons
- Costs may accumulate with extensive usage.
- Requires familiarity with the Azure ecosystem.
User Experience
Users find Microsoft Azure Machine Learning convenient for its integration with Azure services and automated machine learning capabilities.
Top 7: PyTorch
PyTorch is an open-source machine-learning library known for its flexibility and deep learning capabilities.
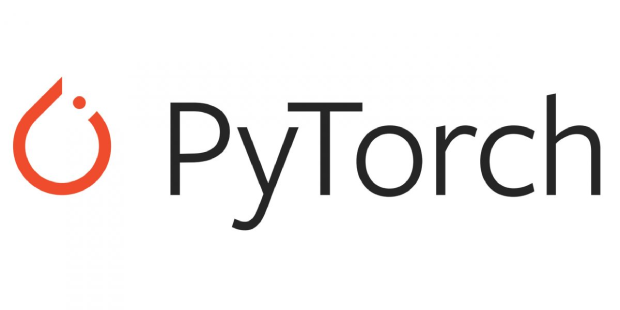
Main Features
- Dynamic computation graph.
- Extensive support for neural networks.
- Pythonic and user-friendly.
- Active community and resources.
Pros
- Flexibility and dynamic computation graph.
- Extensive deep learning support.
- Pythonic and easy to use.
- Active community and resources.
Cons
- The steeper learning curve for deep learning beginners.
- It is not as suited for production deployments.
User Experience
PyTorch is favorite generative ai for data analytics among researchers and developers for its flexibility and extensive deep-learning capabilities.
Top 8: IBM Watson Analytics
IBM Watson Analytics is a cognitive analytics platform that leverages AI and natural language processing to simplify data analysis.
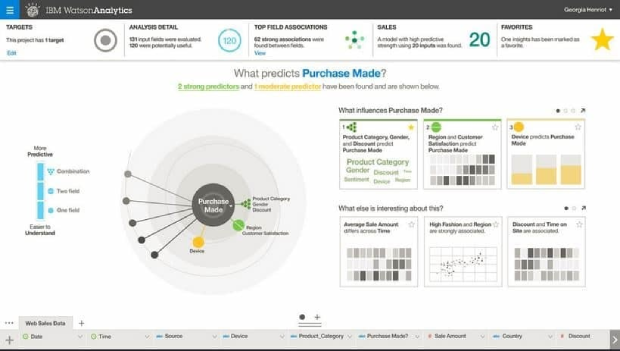
Main Features
- Natural language querying.
- Predictive analytics.
- Data exploration and visualization.
- Automated data preparation.
Pros
- User-friendly interface.
- Natural language querying.
- Predictive analytics capabilities.
- Automated data preparation.
Cons
- Limited customization options.
- Pricing may not be suitable for small businesses.
User Experience
IBM Watson Analytics is commended for its user-friendly approach to data analysis, making it accessible to a wider audience.
Top 9: H2O.ai
H2O.ai is an open-source AI platform that specializes in machine learning and predictive analytics.
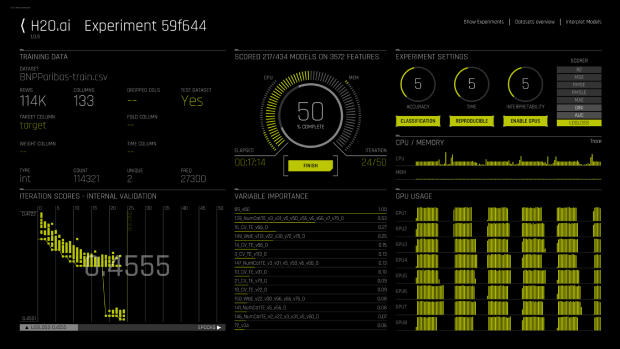
Main Features
- AutoML capabilities.
- Scalable and distributed processing.
- Support for various machine learning algorithms.
- Integration with popular data science tools.
Pros
- AutoML streamlines model building.
- Scalable and distributed processing.
- Extensive machine learning algorithm support.
- Integration with popular data science tools.
Cons
- It may require some technical expertise.
- Learning curve for beginners.
User Experience
Users appreciate H2O.ai for its AutoML capabilities and extensive support for machine learning algorithms.
Part 3: How to Use AI for Data Analysis
By adhering to these steps, data analysts can tap into the capabilities of AI tools, allowing them to acquire valuable insights, make well-informed decisions, and foster innovation and expansion:
Establish Clear Objectives
Begin by definitively outlining the goals of your data analysis project. Identify the specific insights or outcomes you aim to achieve through the utilization of AI-driven analysis.
Data Collection and Preparation
Collect pertinent data from various sources and ensure that it is both clean and properly structured, rendering it suitable for analysis. This phase may encompass activities such as data cleansing, transformation, and standardization.
Select Appropriate AI Techniques
Determine which AI techniques and algorithms align best with your analysis objectives. Contemplate the utilization of machine learning algorithms, deep learning models, natural language processing, or computer vision techniques, depending on the nature of your data and the requisites of your analysis.
Model Training and Evaluation
Employ labeled or historical data to train your AI models. Employ appropriate evaluation metrics to assess the performance of the model and, if necessary, iterate on the training process.
Feature Extraction and Engineering
Extract pertinent features from the data or create new features to enhance the predictive capabilities of the model. This phase may encompass activities like dimensionality reduction, feature scaling, or the generation of novel variables.
Model Deployment
Implement the trained AI models within a production environment, where they can analyze new incoming data. Ensure seamless integration of the models with your existing infrastructure and systems.
Interpret and Validate Results
Scrutinize the outcomes of the AI models and decipher their implications. Validate the findings by cross-referencing them with domain knowledge and conducting statistical tests or validation procedures to guarantee precision and trustworthiness.
Communicate and Visualize Insights
Clearly present the insights and discoveries using data visualization techniques. Utilize charts, graphs, and dashboards to convey intricate information effectively to stakeholders.
Continuously Enhance and Refine
Regularly observe and update your AI models to accommodate evolving data patterns and business requirements. Integrate feedback from users and stakeholders to refine the analysis process continually.
Ethical Considerations
Take into account ethical considerations and potential biases in both the data and AI models. Uphold principles of privacy, fairness, and transparency in the handling and analysis of data.
Part 4: FAQ
Q1: Is there an AI tool for graph data analysis?
Yes, there are AI tools like Neo4j and Gephi that specialize in graph data analysis, helping users uncover patterns and insights within interconnected data.
Q2: Do you know the best AI tool for Excel data analysis?
Microsoft Power BI and Tableau are popular choices for Excel data analysis, offering advanced visualization and analytical capabilities.
Q3: Is there an AI for data visualization?
Yes, many AI-driven tools like Tableau, Power BI, and Google Data Studio enhance data visualization through automation and interactive features.
Conclusion
In 2023, the landscape of AI for data analytics is increasingly powered by AI, offering data professionals a plethora of innovative tools to choose from. Whether you're looking to streamline PDF summarization with Tenorshare AI - PDF Tool AI is revolutionizing the way data is analyzed. The key is to match your specific data analysis needs with the right tool's capabilities. As we conclude this exploration of the best AI tools for data analytics, we recommend Tenorshare AI - PDF Tool for its user-friendly interface, multilingual support, and efficient PDF summarization capabilities. It exemplifies the potential of AI in simplifying complex tasks and enhancing productivity in the realm of data analysis.